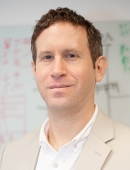
Yuval Itan, PhD
About Me
Dr. Yuval Itan is an Associate Professor in the Department of Genetics and Genomic Sciences, a core member of The Charles Bronfman Institute for Personalized Medicine, and a member of Mindich Child Health and Development Institute, at the Icahn School of Medicine at Mount Sinai in New York City.
The main focus of the Itan lab is investigating human disease genomics for enhancing precision medicine, by developing new machine learning and computational methods to detect disease-causing variants and genes in next generation sequencing data of patients, and by performing cases-controls genome- and phenome-wide studies of patient cohorts across diverse human populations to identify new genetic etiologies of human diseases.
The Itan lab applies and combines diverse approaches across computer science and biology, including machine learning, natural language processing, bioinformatics, statistical genomics, modelings and simulations, and population genetics.
Language
Position
Research Topics
Bioinformatics, Biomedical Informatics, Biomedical Sciences, Biostatistics, Cardiovascular, Clinical Genomics, Computational Biology, Computer Simulation, Coronavirus, Epidemiology, Evolution, Gastroenterology, Gene Discovery, Genetics, Genomics, Immune Deficiency, Infectious Disease, Inflammatory Bowel Disease (IBD), Mathematical Modeling of Biomedical Systems, Mathematical and Computational Biology, Neural Networks, Obesity, Parkinson's Disease, Personalized Medicine, Proteomics, Sequence Alignment, Systems Biology, Technology & Innovation, Theoretical Biology, Translational Research
Multi-Disciplinary Training Areas
Artificial Intelligence and Emerging Technologies in Medicine [AIET], Genetics and Genomic Sciences [GGS]
About Me
Dr. Yuval Itan is an Associate Professor in the Department of Genetics and Genomic Sciences, a core member of The Charles Bronfman Institute for Personalized Medicine, and a member of Mindich Child Health and Development Institute, at the Icahn School of Medicine at Mount Sinai in New York City.
The main focus of the Itan lab is investigating human disease genomics for enhancing precision medicine, by developing new machine learning and computational methods to detect disease-causing variants and genes in next generation sequencing data of patients, and by performing cases-controls genome- and phenome-wide studies of patient cohorts across diverse human populations to identify new genetic etiologies of human diseases.
The Itan lab applies and combines diverse approaches across computer science and biology, including machine learning, natural language processing, bioinformatics, statistical genomics, modelings and simulations, and population genetics.
Language
Position
Research Topics
Bioinformatics, Biomedical Informatics, Biomedical Sciences, Biostatistics, Cardiovascular, Clinical Genomics, Computational Biology, Computer Simulation, Coronavirus, Epidemiology, Evolution, Gastroenterology, Gene Discovery, Genetics, Genomics, Immune Deficiency, Infectious Disease, Inflammatory Bowel Disease (IBD), Mathematical Modeling of Biomedical Systems, Mathematical and Computational Biology, Neural Networks, Obesity, Parkinson's Disease, Personalized Medicine, Proteomics, Sequence Alignment, Systems Biology, Technology & Innovation, Theoretical Biology, Translational Research
Multi-Disciplinary Training Areas
Artificial Intelligence and Emerging Technologies in Medicine [AIET], Genetics and Genomic Sciences [GGS]